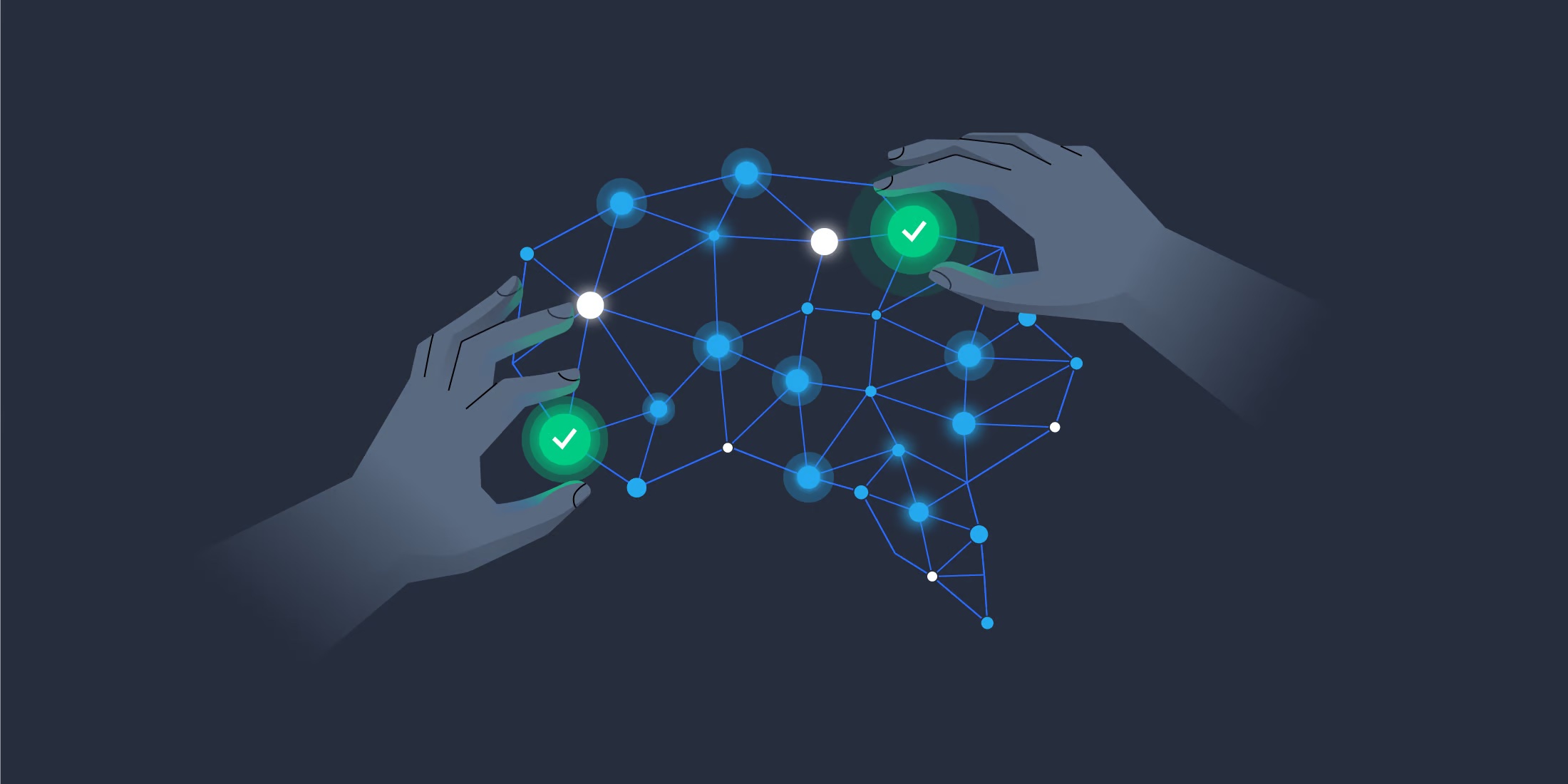
The Transition from Product Manager to AI/ML Product Manager
While Senior Product Managers and AI/ML Senior Product Managers share many responsibilities, the latter requires a more specific set of skills and a deep understanding of artificial intelligence and machine learning. Here are ten differences that delineate the two roles:
- Understanding of AI/ML: Unlike traditional Senior Product Managers, AI/ML Senior Product Managers need a fundamental understanding of AI and ML concepts. They don’t necessarily need to code algorithms but should understand how they work to make informed decisions about product features and improvements.
- Data-Driven Decision Making: While all Product Managers should be data-driven, AI/ML Product Managers often work directly with complex datasets and need a deeper understanding of statistical analysis and data modeling to guide the product’s direction.
- Close Collaboration with Data Scientists: AI/ML Product Managers frequently work in close collaboration with data scientists, ML engineers, and data engineers. They need to understand the language and workflows of these roles better than a traditional Product Manager might.
- Algorithm Evaluation: AI/ML Product Managers should be able to understand and discuss the evaluation of machine learning models. They need to comprehend terms like precision, recall, AUC-ROC, and understand how changes to these metrics can impact the user experience.
- Ethics and Bias Considerations: AI/ML Product Managers need to be keenly aware of the ethical implications of their products. They should understand how biases can be inadvertently built into ML models and work to mitigate these risks.
- Product Experimentation: AI/ML Product Managers often need to use different techniques for A/B testing or experimentation due to the complexity of AI/ML products. They need to account for factors like concept drift and model interpretability when testing new features or improvements.
- Model Deployment and Monitoring: Unlike traditional software products, AI/ML models require ongoing monitoring and maintenance after deployment. AI/ML Product Managers need to understand this lifecycle and plan for it in their product roadmap.
- Data Privacy: AI/ML Product Managers should be more familiar with data privacy laws and regulations, as AI/ML models often rely on large amounts of potentially sensitive data.
- Technical Liaison: An AI/ML Product Manager often acts as the bridge between highly technical AI/ML teams and other parts of the business. They need to translate complex AI/ML concepts into understandable terms for stakeholders, more so than traditional Product Managers.
- Long-term Vision for AI: AI/ML Product Managers are tasked with envisioning how AI can shape the future of their products. They need to balance short-term gains from AI/ML features with a long-term strategic vision for how AI can provide a competitive edge.
While the transition from a Senior Product Manager to an AI/ML Senior Product Manager can be challenging, it offers an exciting opportunity to shape the future of products at the cutting edge of technology. It requires a mix of technical depth, strategic thinking, and excellent communication skills to succeed.